Downhole Fracture Diagnosis and Mud Loss Control Technologies Bases on Neural Network Algorithm
-
摘要: 在钻井过程中,常常钻遇不同宽度的井下地层裂缝。钻遇裂缝时容易发生钻井液漏失现象,甚至发生钻井液失返现象,严重影响了安全、高效钻井。目前裂缝封堵的方法常存在封堵成功率不高、堵漏承压能力低的问题,其中一个重要的原因是对井下地层的裂缝宽度等特征认识不清。基于地层裂缝产生的岩石力学机理,确定影响裂缝宽度关键的6个力学和工程因素,并利用神经网络计算的非线性、大数据特点建立了井下地层裂缝宽度的分析模型,模型包含输入层、输出层和3个隐藏层。通过该模型诊断井下裂缝宽度,提高了计算精度,平均误差仅为2.09%,最大误差为5.88%,解决钻井现场仅凭经验判断裂缝误差较大和依靠成像测井成本较高的问题。同时根据神经网络模型诊断得到的裂缝宽度优化堵漏材料的粒径配比,提高了裂缝内的架桥封堵强度和架桥的稳定性,封堵层的承压能力达到12.8 MPa,反向承压能力达到4.5 MPa。现场堵漏试验最高憋压10 MPa,经过封堵作业后大排量循环不漏,达到了裂缝性地层高效堵漏的目的,堵漏一次成功。Abstract: Formation fractures of different widths are always met during drilling, resulting in partial mud losses or even lost return which seriously affect drilling in a safe and efficient manner. Presently, the success rate of controlling mud losses into fractures is not high, and the fractures plugged have low pressure bearing capacities. One cause to these problems is the lacking of understanding of the widths of the fractures into which mud is lost. In this paper, rock mechanics mechanisms of formation fracture generation are used as the bases to determine the 6 mechanical and engineering factors affecting the width of fractures produced downhole. Using the nonlinearity and big data analysis natures of neural network computation a model for analyzing the widths of downhole formation fractures was established, which included input layer, output layer and 3 hidden layers. Using this model, the calculation precision of diagnosing the widths of downhole fractures was improved to an average error of only 2.09% and the maximum error of 5.88%, resolving the problem of predicting fracture width through experiences which is inaccurate or through imaging logging which is expensive. The widths of fractures calculated with the model were also used to optimize the particle size distribution of plugging additives, helping improve the strength and stability of bridging inside the fractures, the pressure bearing capacity of the plugged fractures was increased to 12.8 MPa and the back-pressure bearing capacity to 4.5 MPa. In field application, the highest pressure squeezed on the plugged fractures was 10 MPa, and the plugged fractures were able to stand large flow rate circulation, indicating that the fractured formations were effectively and successfully plugged.
-
Key words:
- Drilling /
- Fracture width /
- Neural network /
- Particle size distribution /
- Mud loss control
-
[1] 方俊伟,吕忠楷,何仲,等. 化学凝胶堵剂承压堵漏技术在顺北3井的应用[J]. 钻井液与完井液,2017, 34(6):13-17.FANG Junwei,LYU Zhongkai,HE Zhong,et al. Application of chemical gel LCM on well Shunbei-3[J]. Drilling Fluid & Completion Fuild,2017,34(6):13-17. [2] 王广财,王丰年,张军,等. 凝胶复合防漏堵漏技术在火焰山腹地表层的应用[J]. 钻井液与完井液,2017, 34(3):49-53.WANG Guangcai,WANG Fengnian,ZHANG Jun,et al. Application of a composite gel lost circulation material in the top section of wells drilled in the central region of the mountain of fire[J]. Drilling Fluid & Completion Fluid, 2017,34(3):49-53. [3] 屈璠,韦西海,吴金星,等. 狮202井区裂缝性地层堵漏技术[J]. 钻井液与完井液,2018,35(2):78-84.QU Fan, WEI Xihai, WU Jinxing, et al.Techniques for mud loss control in drilling fractured formations in Shi-202 well zone[J].Drilling Fluid & Completion Fluid, 2018,35(2):78-84. [4] 黄荣樽.水力压裂裂缝的起裂和扩展[J].石油勘探与开发,1981,8(5):62-74.HUANG Rongzun. Cracking and expansion of hydraulic fracturing cracks[J]. Petroleum Exploration and Development, 1981, 8(5):62-74. [5] 金衍,陈勉,张旭东.天然裂缝地层垂直井水力裂缝起裂压力模型研究[J].石油学报,2005,26(6):113-115.JIN Yan, CHEN Mian, ZHANG Xudong. Research on hydraulic fracture initiation pressure model of vertical well in natural fracture formation[J]. Acta Petrolei Sinica, 2005, 26(6):113-115. [6] HAIMSON B, FAIRHURST C. Initiation and extension of hydraulic fractures in rocks[J]. Society of Petroleum Engineers Journal, 1967, 7(3):310-318. [7] 冯夏庭, 贾民泰. 岩石力学问题的神经网络建模[J]. 岩石力学与工程学报, 2000(S1):1030-1033. FENG Xiating, JIA Mintai. Neural network modeling of rock mechanics problems[J].Chinese Journal of Rock Mechanics and Engineering,2000(S1):1030-1033. [8] 张盛, 王启智. 神经网络在研究岩石断裂韧度尺寸效应的应用[J]. 四川大学学报(工程科学版), 2005, 37(3):37-41. ZHANG Sheng, WANG Qizhi. Application of neural network in studying the size effect of rock fracture toughness[J].Journal of Sichuan University(Engineering Science Edition), 2005, 37(3):37-41. [9] PERKINS T K,KERN L R. Widths of hydraulic fractures[J].Journal of Petroleum Technology, 1961, 13(9):937-949. [10] ALBERTY M W, MCLEAN M R. Fracture gradients in depleted reservoirs-drilling wells in late reservoir life[C]//SPE/IADC drilling conference. Society of Petroleum Engineers, 2001. -
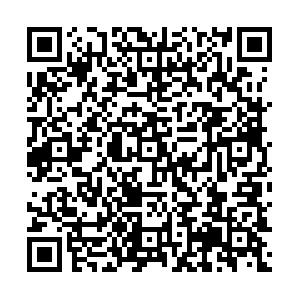
计量
- 文章访问数: 537
- HTML全文浏览量: 165
- PDF下载量: 114
- 被引次数: 0